Injuries can derail an athlete’s career and the trajectory of a franchise. Until recently, injuries were thought of as the cost of doing business. Over the last decade, professional sports franchises have poured more resources into keeping their athletes healthy than ever before. Much of the conversation has since focused on Load Management. Teams are trying to maintain sufficient “chronic” workloads, while reducing “acute” spikes in load.
Though reducing spikes in workload may be beneficial, alone it is insufficient.
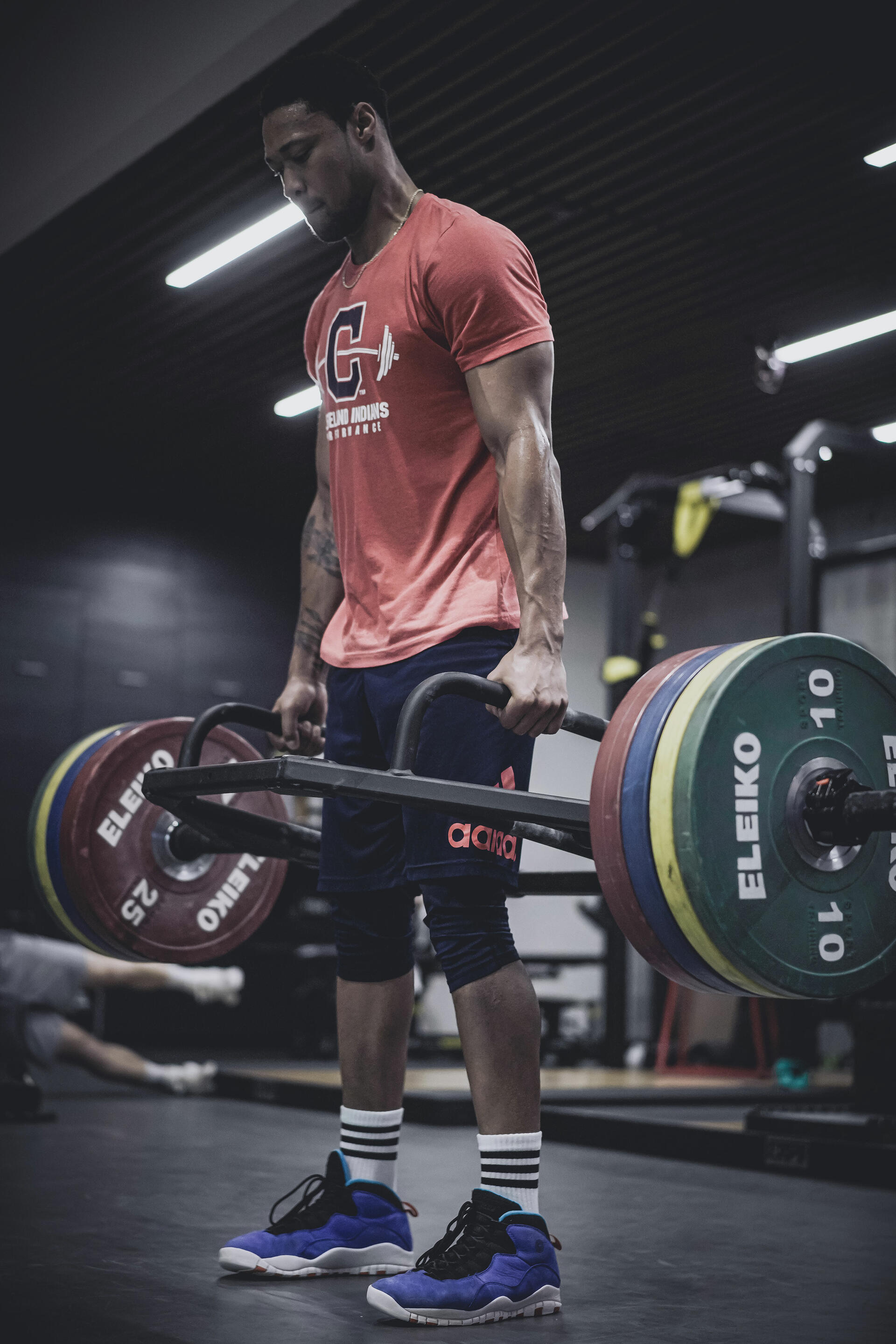
How does P3 address the injury problem?
While the aim of Load Management is to restore an athlete’s physical baseline, our philosophy is that more developed athletes are generally more resilient – suggesting that work should be done to improve an athlete’s physical, mechanical baseline. This is where we believe we can contribute to the field: by studying the data at our disposal relentlessly to examine any relationships between movement strategy in the lab and injury outcomes on court or on-field, all for the purposes of shifting an athlete’s mechanical baseline. If we can detect an issue before it arises, it’s our job to intervene.
Injury Pattern Detection
P3 collects hundreds of data points per second as athletes perform sport-specific movements in our lab. We have performed thousands of assessment trials and the rate of growth of our database continues to accelerate. The sheer magnitude of data requires P3 engineers to use pattern recognition to help sort through it all.
Machine learning (ML) is an application of artificial intelligence (AI) that provides systems the ability to learn and iterate as they get exposed to new data – for each new athlete that we assess, our algorithms should get that much smarter. While ML has been around for years, it’s become increasingly utilized in sport with the introduction of big data.
Video file
We leverage machine learning for applications that are going to allow us to identify windows of opportunity for an athlete’s physical development, or hunt for mechanics that may predispose an athlete to injury.
This has helped our sport scientists better understand the interplay between how various physical parameters and movement strategies can increase or decrease an athlete’s risk of injury, allowing us to stratify prospective risk of injury immediately following an assessment. Once an area is targeted as high risk, we tailor specific exercises to address the underpinning mechanics. Combining human expertise with ML systems provides better results than either human or machine alone when it comes to understanding injury risk.
Case Study
How P3 developed a model that is capable of identifying 6 out of the next 10 athletes who will suffer a prospective non-contact knee injury in the NBA
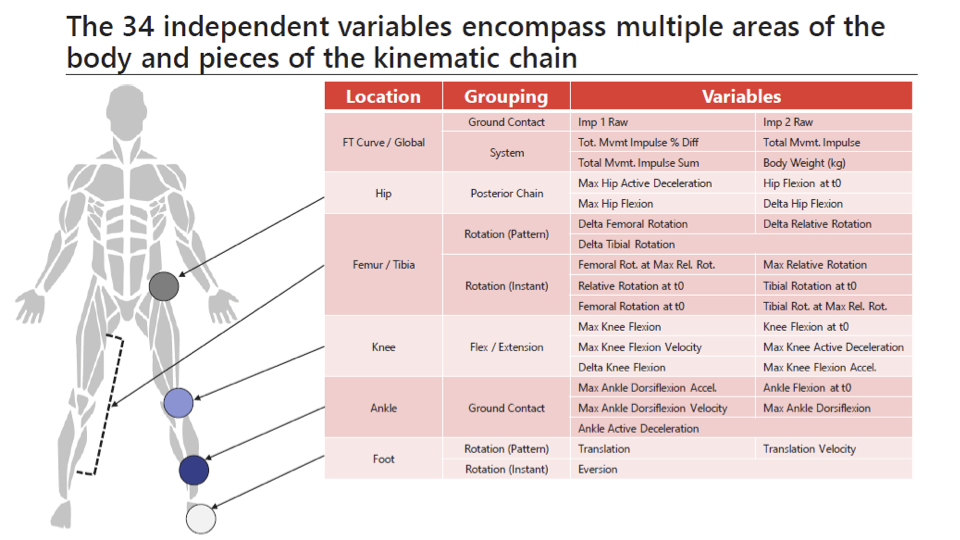
We began collecting 3D Motion Capture and Force Plate data for this study in the summer of 2013, with the intent to better understand the underlying biomechanical factors that contribute to acute knee injuries – defined here as non-contact, traumatic injury to the ACL, MCL, or meniscus. Can biomechanics and machine learning be used to not only better identify athletes who are at risk, but also provide course corrective feedback to mitigate that risk?
Over 400 professional basketball players volunteered to partake in this study, generating approximately 500 variables per athlete, per assessment – the largest of its kind in elite basketball. Following an assessment, injury outcomes were tracked over 2 years by P3 staff.
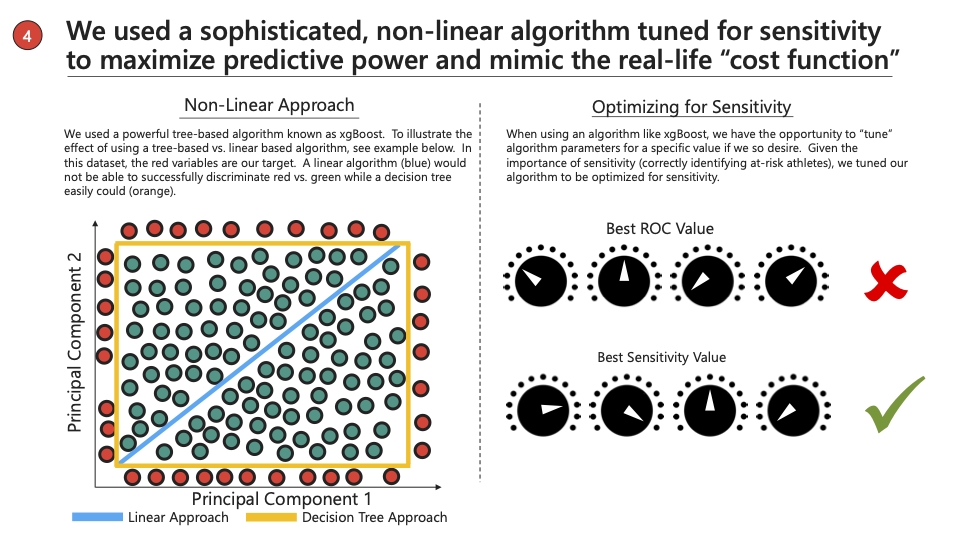
We built a binary classification model using gradient boosted decision trees and pre-processing steps – SMOTE oversampling alongside stratified, repeated k-fold cross validation - designed to combat “class-imbalance” issues in our dataset. Our model was tuned for sensitivity to reflect the “real-life” cost function of an acute injury to the knee. Identifying true positives lives at the heart of this study.
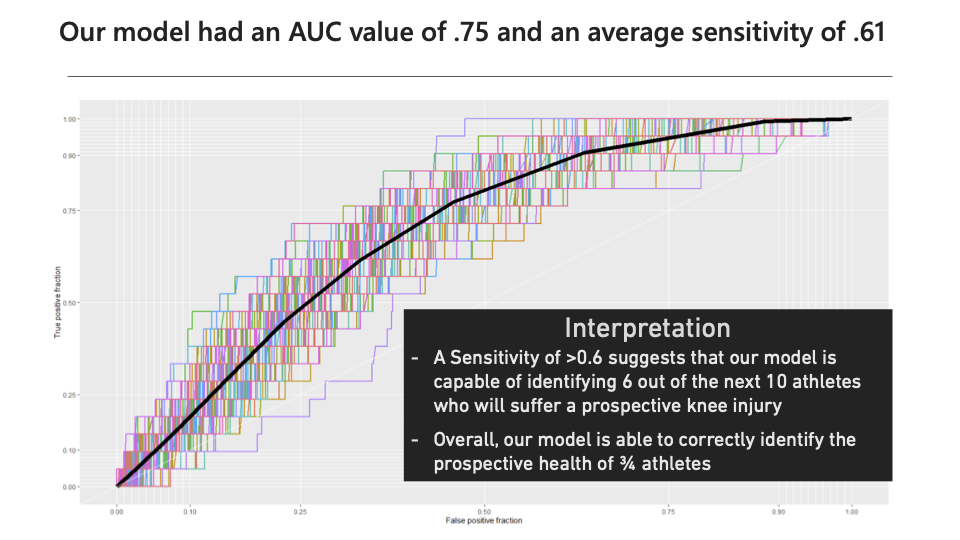
Our model possesses an AUC of 0.75, with a sensitivity greater than 0.6. In practical terms, our model should prospectively identify 6 out of 10 athletes who will suffer an acute knee injury – and, most importantly, provide the opportunity to intervene.
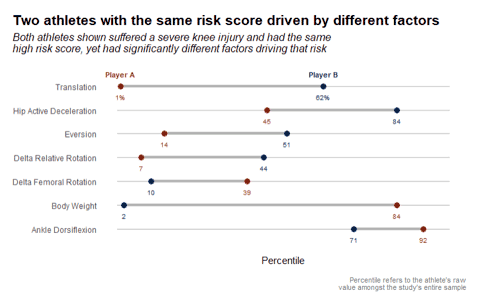
By extracting feature importance from our model, we can unpack the unique factors that drive each athlete’s risk of injury. While two athletes may have a similar risk score, the factors driving their scores may be different: their risk mitigation strategies should reflect this reality. If we do our job well, we should be able to mitigate certain injury risks before they turn problematic.
These insights can help performance staffs working in elite basketball to better identify underlying risk factors and address them prior to injury. As the discussion moves from Load Management and workload reduction to preemptive development, we can increase the likelihood that the NBA’s best athletes see out their full potential.